AI-RAPTOR
Full project title: Artificial Intelligence for Radiological Assistance for Pulmonary and ThOracic analysis in tumor Recognition and screening.
Project period
Start: January 2023
End: December 2025
Approximately 60% of lung cancer cases are diagnosed at advanced stages, making lung cancer the leading cause of cancer-related deaths worldwide. It is therefore crucial that methods for earlier detection are developed to improve lung cancer survival.
Recent studies have shown that a screening using low-dose computed tomography (also called low-dose CT scans or LDCT) of high-risk individuals can effectively reduce the mortality of lung cancer patients by offering early detection of signs of cancer.
The health authorities in Denmark are planning to implement a national lung cancer screening programme in 2024 to enhance early detection, but introducing a CT-based screening programme will increase the workload by approximately 100.000-150.000 additional CT scans per year and exacerbate the existing shortage of specialised radiologists. Currently, the time from CT to diagnosis is approximately 24 days, but with a screening programme based on the proposed inclusion criteria from the NELSON trial, the Danish hospitals would need to have up to four times as many specialised radiologists compared to today to be able to maintain this timeframe.
Artificial intelligence (AI) technologies might offer an answer to these challenges. AI-based algorithms have shown themselves to be quite useful in areas such as medical imaging, and a recent review has found that algorithms are capable of detecting lung cancer. Despite this, it is still necessary to evaluate the clinical utility, safety, method of reporting as well as the feasibility of implementing AI technology in clinical practice.
Aim
AI-RAPTOR is a PhD study by Frederik Andersen and its overall aim is to develop, train and validate an AI algorithm that can detect potentially malignant pulmonary findings on the low-dose CT scan and thus aid clinicians in the diagnostic process.
The algorithm will, once implemented, perform the initial analysis of the images from the CT scan and combine these findings with patient data such as age, smoking habits and medical history to suggest a diagnosis. The specialised lung radiologist will then review the findings and use these for decision support to reach a diagnosis.
The proposed AI-based clinical decision support tool is expected to cut down the time frame from CT scan to diagnosis without compromising the quality of care, as the solution allows for a broader set of data to be analysed and included in the diagnostic process.
The AI-RAPTOR project will be divided into three sub-studies:
- A systematic review of current literature regarding the use of AI in pulmonary findings in low-dose CT screenings. This is to ensure optimal background knowledge for the design of the best possible algorithm.
- Development, training and validation of the algorithm
For this study we will create a dataset for training the algorithm, consisting of approximately 5000 anonymised CT scans from patients previously referred for a diagnostic lung cancer workup (“Lungepakken”) and the Danish Lung Cancer registry. The dataset will contain both normal pulmonary findings (no pathology) as well as benign and malignant pulmonary nodules (confirmed by histopathology). Once the refining and training process is completed, the performance (sensitivity and specificity) of the algorithm will be compared and validated against the evaluation made by the radiologists (all included CT scans have written reports) and the histopathology (biopsy or surgery). - Validating and assessing the clinical usefulness and feasibility of the developed algorithm in comparison to current clinical practice
In a clinical setting involving both radiologists and the diagnostic unit (Department of Respiratory Medicine), the algorithm will be subjected to a highly relevant potential screening population containing 15.000 anonymised CT scans available from the Danish Cardiovascular Screening Trial (DANCAVAS) performed from 2014 to 2017. This will facilitate a descriptive analysis of the cohort and the incidence of suspicious pulmonary nodules in a simulated clinical setting and the feasibility. The workflow with integrated algorithm will be compared to the current workflow in terms of accuracy and feasibility. The performance of the algorithm will be compared and validated against the evaluation of expert thoracic radiologists and radiology residents using a randomly selected subset of CT scans, hereby investigating the performance.
Participants
- Department of Thoracic, Cardiac and Vascular Surgery, Odense University Hospital
- Department of Respiratory Medicine, Odense University Hospital
- Department of Radiology, Odense University Hospital
- Regional IT, Region of Southern Denmark
- The Maersk Mc-Kinney Moller Institute, University of Southern Denmark
AI consultant and PhD student Simon Lyck Bjært Sørensen is lead developer on the project.
Funding
The PhD study AI-RAPTOR has received funding from the Independent Research Fund Denmark (DFF) and the local Innovation Fund in the Region of Southern Denmark.
Read more about AI-RAPTOR
Read a news article about the project from the University of Southern Denmark
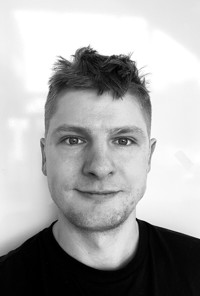
Frederik Andersen
MD, PhD Student
Odense University Hospital, Department of Thoracic, Cardiac and Vascular Surgery
frederik.andersen@rsyd.dk
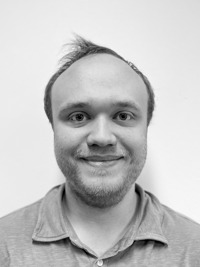
Simon Lyck Bjært Sørensen
PhD student
Odense University Hospital, Department of Thoracic, Cardiac and Vascular Surgery
simon.lyck.bjaert.sorensen@rsyd.dk
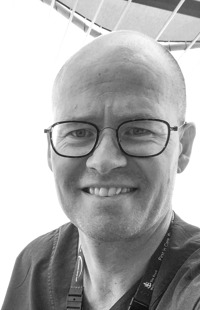
Michael Stenger
MD, PhD, Thoracic Surgeon, Associate Professor
Odense University Hospital, Department of Thoracic, Cardiac and Vascular Surgery
michael.stenger@rsyd.dk
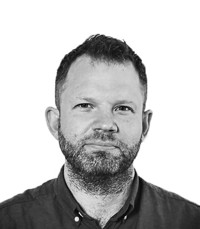
Benjamin S. Rasmussen
Head of Clinical Research - MD (Radiologist), Associate Professor
Centre for Clinical Artificial Intelligence (CAI-X). Odense University Hospital, Department of Radiology
(+45) 2434 1749 benjamin.rasmussen@rsyd.dk